I am echoing my own writing on the next article as a opening here, in which I will state that I don’t like repetition, but some readers can get benefit or hope from it, depending on the information being repeated, in this regard the next article is a republishing with additions of my Heal Thyself article.
Ironically a news piece today made me want to republish but also add thoughts to it, the news is this specific one, which sent me into a laughing fit. Larry Ellison of Oracle.
If you are a long time readers, you shouldn’t be surprised by the slightest since both me and a few others stated, since the announcement of using the novel platform mRNA as a vaccine platform, the original usage was a cancer vaccine. Below the reproduction of a older article, necessary context for what I will write here, which will be below a divider line.
Before going further another explanation. An algorithm is a set of instructions, from simple to incredibly complex, where a computer/program will do what you ask. You give it data, and if you give the same data 10 times, you will get the same result 10 times. An AI is adaptable, it can contextualize, it can change “midway”, and it can learn. This was heavily simplified, but the distinction here is necessary.
A specific news clip that my brain instantly committed to memory was an early interview from Pfizer’s CEO, in a moment where the interviewer asked how Pfizer came up with the vaccine so incredibly fast, Bourla replied: “We used an algorithm, and after some time the machine gave us the result”. I tried very hard to find the clip, but couldn’t and can’t spend 6-12 hours going through hundreds of useless videos, but I did find something “better”.
Pfizer Discusses Use of Supercomputing and AI for Covid Drug Development
Over 16 months ago, Pfizer achieved a historic scientific moonshot — the unprecedentedly swift development and authorization of a novel vaccine for a novel virus using methods that hitherto had not been used in approved drugs at scale. Throughout the pandemic, nearly every public research supercomputer pivoted to some form of Covid research, but the pharmaceutical giants were characteristically cagey about their use of advanced technologies for vaccine and therapeutic development.
“Pfizer is applying digital data and AI across the entire value chain,” Fonseca said, “making our work faster and easier and enhancing every aspect of our business. We’re driving this end-to-end innovation with three strategic priorities in mind: first, to improve patient health outcomes; second, to bring medicines to patients faster; and third, to fuel tomorrow’s breakthrough therapies.”
“In research and discovery, we leveraged supercomputing, AI and machine learning to accelerate the identification of the most promising target compounds…”
The following quote comes from this Mckinsey article, both of the article above and the one below are worth reading. This last one is more of an observation and extrapolation case study, you can deduce how Pfizer and many other big pharmaceutical companies hedged their bets and acquired so many “peculiar” biotech companies to treat“rare” conditions.
Siddhartha Chadha: What difference did digital make when Pfizer faced the urgent need to develop a COVID-19 vaccine?
Lidia Fonseca: Simply put, digital enabled us to develop a safe, effective vaccine in record time, without cutting corners.
I will leave the arguments for or against Algocracy (a state we definitely live on, and have for years now) for another time or better writers, but the point I am making here, and a question people from all walks of life, from the average person to the some of the most talented scientists had was “Why they chose the Spike Protein as a vaccine target ?”
Simply put, their algorithms were either trained on insufficient data or badly designed from the group up, perhaps both at that point in time, chose the Spike precisely because depending on how you design your software or algo, the Furin Cleavage Site lights up like a Christiams tree. Until ChpatGPT 3.5 and 4, most AI was not that sophisticated, in the near future such systems will be defined as either “sophisticated algorithms” or “very rudimentary AI tools”, and as such the software picked up the Spike Protein because of that. (or it was DARPA’s ADEPT software ? A conspiracy contemplation for your Friday).
As a matter of fact, to demonstrate how powerful properly designed algorithm, especially aided by properly trained AI, can be, here is a Nature paper published by Chinese scientists, with the help of the Baidu AI department (Baidu being one of the biggest tech companies in China, focused on AI now).
Algorithm for Optimized mRNA Design Improves Stability and Immunogenicity
On both COVID-19 and varicella-zoster virus mRNA vaccines, LinearDesign substantially improves mRNA half-life and protein expression, and dramatically increases antibody titer by up to 128× in vivo, compared to the codon-optimization benchmark. This surprising result reveals the great potential of principled mRNA design, and enables the exploration of previously unreachable but highly stable and efficient designs. Our work is a timely tool not only for vaccines but also for mRNA medicine encoding all therapeutic proteins (e.g., monoclonal antibodies and anti-cancer drugs (7, 8)).
Another reproduction of a old article.
From this line forward, it is new.
The algorithm above was designed by Chinese researchers with the help of Baidu AI division, and their simple yet elegant algorithm made substantial improvements on the mRNA stability. To add another layer of complexity here, a recent paper titled “Nanocarrier imaging at single-cell resolution across entire mouse bodies with deep learning” made the rounds on social media, for all the wrong reasons.
Here the authors using a quite creative deep learning approach develop al algorithm that can measure, at a single cell level the distribution of mRNA and AAV2 (a newcomer and favorite in the gene therapy world), they found the obvious that the mRNA Spike Protein gets to the heart, liver, spleen, bla bla bla. The focus here should have been on their open sourced algorithm and deep learning approach. Why ?
Because even before this Machine Learning — Artificial Intelligence craze after ChatGTP 3.5 was made public, I have stated that they were using ML/AI approaches, and the biggest hurdle was data, and the most likely outcome was pushing the mRNA was a data gathering approach. Machine Learning and current Aritifical Intelligence are massive pattern recognition machines, working the distributions and probabilities, and sometimes averages of data at a scale we have never seen before.
Machine Learning needs an absurd amount of data to learn, but it isn’t like us, who can infer high levels of complexity from little data, it needs millions and millions of examples to understand the patterns, relationships between the data, and learn. And such for a short while the ML/AI was facing a data wall, they needed more data, but there was no more data to gather or scrape, so they invested billions into creating high quality synthetic data (data that mimics, perfectly, high quality human data), and thus pushed models further.
Up until late 2020, mRNA had no data, and well, if you want to use Machine Learning to improve your technology, you need data. In fact, you could argue you need a entire world worth of biological data. Billions of injected doses later, we’ve gifted AI systems a deluge of pharmacokinetic, immunogenic, and cellular-distribution data. If you always wanted to have a hypothetical example of how the SARS-CoV-2 vaccine was made, here is a (entirely fake) algorithm. Download the image to zoom in if you are curious, had to add another image otherwise it was excessively large, first one is the entire algo.

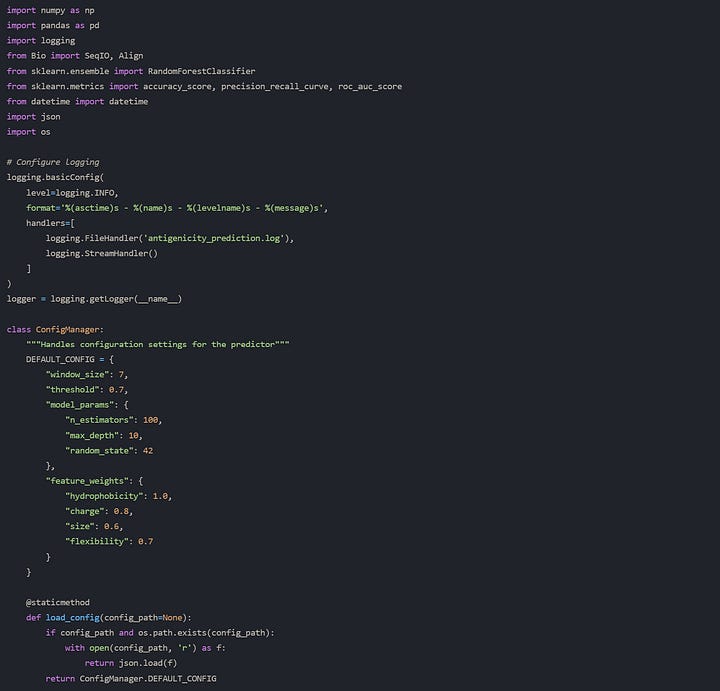
This is news not solely because of Ellison taking the place of Bill Gates pushing new tech, but because together with the new Trump administration, Oracle, OpenAI and SoftBank announced a new AI-centered project, named Stargate. A 500 billion dollars investment in AI infrastructure with 100 of these Bi being deployed this year alone. I will save my disagreements with all the players involved here and their economics for another day.
While I personally have substantial and colossal disagreements with a lot of what is going on in ML/AI, and especially with OpenAI’s instance and approach, the truth is we now have large amounts of data, we will soon have the compute (the infrascture and hardware to make AI think for longer and “harder”), and also models smart enough to do this.
Personalized mRNA vaccines are nothing new, this trend has been in the news for almost a year. Here a Chinese team combining a personalized mRNA vaccine with a monoclonal to treat advanced solid tumors. A British man here. Russia will offer its mRNA cancer vaccine free of charge, which they used AI to develop.
It was always the next step, and there is a promise there, although the catch is yet to be figured out even if it is personalized, and toxicities and everything else is figured out, although purity wise, personalized mRNA is bench quality, meaning highly pure, since you make small batches, not in bioreactors of 50 liters.
This was inevitable, the presence of AI in almost every aspect of our lives was, is and will remain inevitable too, the question is who sits at the silicon throne, who will owns and dictate the path of AI. A handful elitistic few, or everyone else ? Such as Anthropic who wants to regulated the development and usage of AI, while doing this.
I will not be surprised if Anthropic is the cause of the cyber pandemic, because their safety facade is falling. Doing some of the most dangerous experiments with advanced AI systems and I KNOW not with the necessary precautions. I guess that is why their founder was in Davos… perhaps it is time to write my Cyberpunk 2077 article.
I appreciate and welcome your support. Thank you !
While AI is incredible and has many usages, it is so confusing and produces so much "slop" (bad quality outputs) because of lack of proper education among the population that instead of me being without a job, people ask me if the AI is right on its takes.
Lol, lmao even, I got that one wrong.
As for Pfizer using AI to design and codon-optimize BNT162b2, there were 2 versions admitted to (maybe there were versions 1-7?)
version 8 which used a BioNTech proprietary algorithm
version 9 which used a published algorithm (Raab et al. 2010)
they decided on version 9.....I have always wondered why